Technology and Engineering
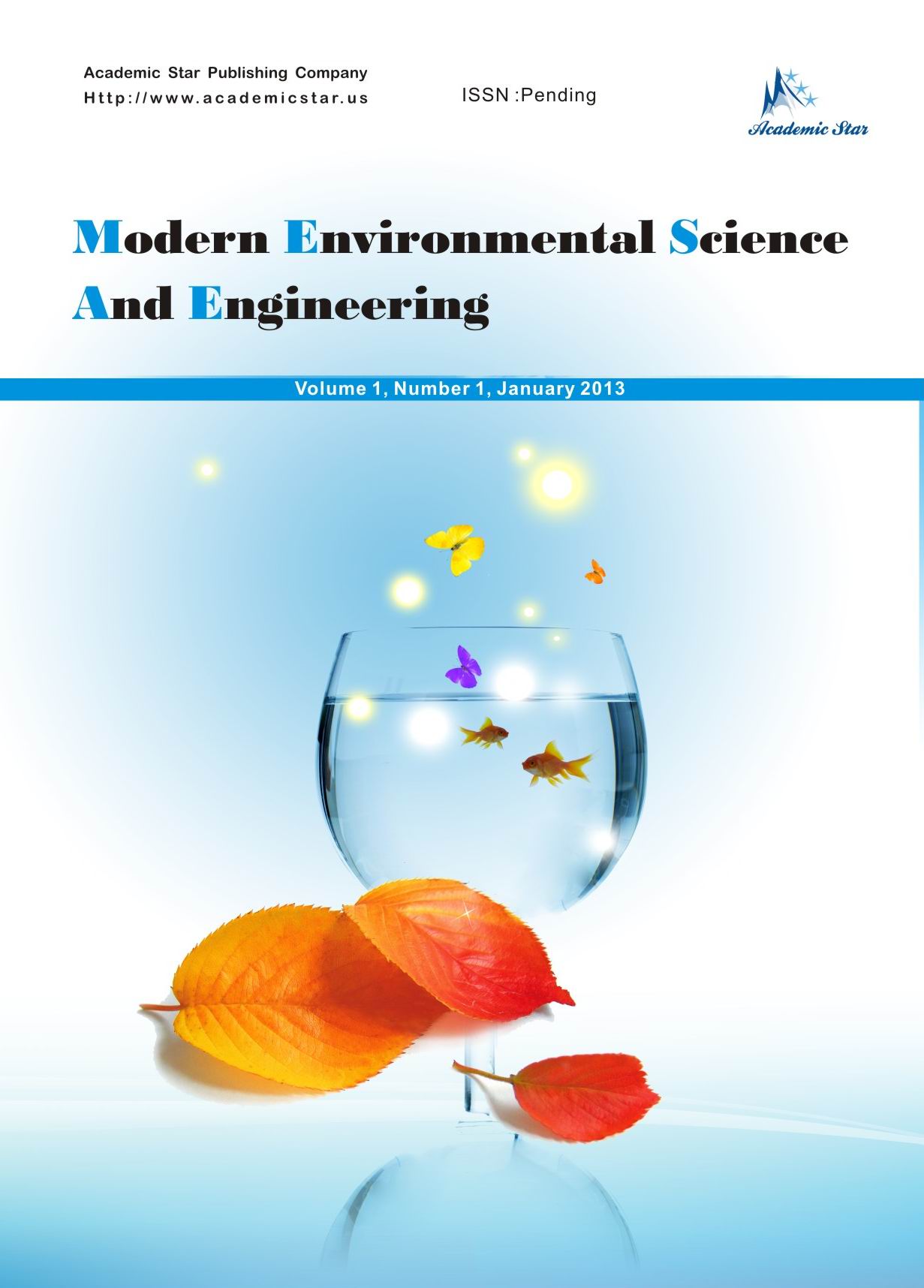
- ISSN: 2333-2581
- Modern Environmental Science and Engineering
Application of Kalman Filter Recurrent Neural Network for Identification and Control of Hydrocarbon Biodegradation Bioprocess
Ieroham Solomon Baruch1, Josefina Barrera Cortes2, and Carlos-Roman Mariaca Gaspar3
1. Department of Automatic Control, Center of Investigation and Advanced Studies, National Polytechnical Institute, Mexico
2. Department of Biotechnology and Bioengineering, Center of Investigation and Advanced Studies, National Polytechnical Institute, Mexico
3. ESIME-IPN, Mexico D.F., Mexico
Abstract: Biological treatment is attractive as a potentially low-cost technology, which converts toxic organic contaminants into CO2 and biomass. Since the 70’s, this technology has been applied for the hydrocarbon degradation, and today, it is considered as the best alternative to clean up polluted soils. For this bioprocess, one challenge is to provide enough O2 and nutrients to enable rapid conversion of contaminants by either indigenous microorganisms or inoculated species. Another challenge is to achieve efficient contact between the active micro-organisms and the contaminants, which may represented a problem with in-situ treatment. An attractive alternative to overcome this problem is to apply a biological treatment in slurry phase using Horizontal Rotating Drum (HRD). Nowadays, semi empirical HRD models, based on the Monod equation, have been developed to predict micro-organism growth as a function of available contaminants concentration. However, as the application of such models requires experimental work for calculating the kinetics parameters involved, so an alternative modeling technique is required. The Kalman Filter Recurrent Neural Network Model (KF RNNM) offers many advantages as the possibility to approximate complex non linear high order multivariable processes, as the biodegradation process is. The KF RNNM has been applied for measurement data filtering and parameters plus state estimation of hydrocarbons biodegradation process, contained in polluted slurry, treated in a rotating bioreactor. Then the KF RNNM is simplified and used to design a Sliding Mode Control (SMC) of two-input two-output high order nonlinear plant. The KF RNNM learning algorithm is the dynamic Backpropagation one (BP). The graphical simulation results of the system approximation, and indirect adaptive neural control, exhibited a good convergence, and precise reference tracking.
Key words: hydrocarbon biodegradation bioprocess, horizontal rotating drum, identification and sliding mode control, Kalman filter recurrent neural network